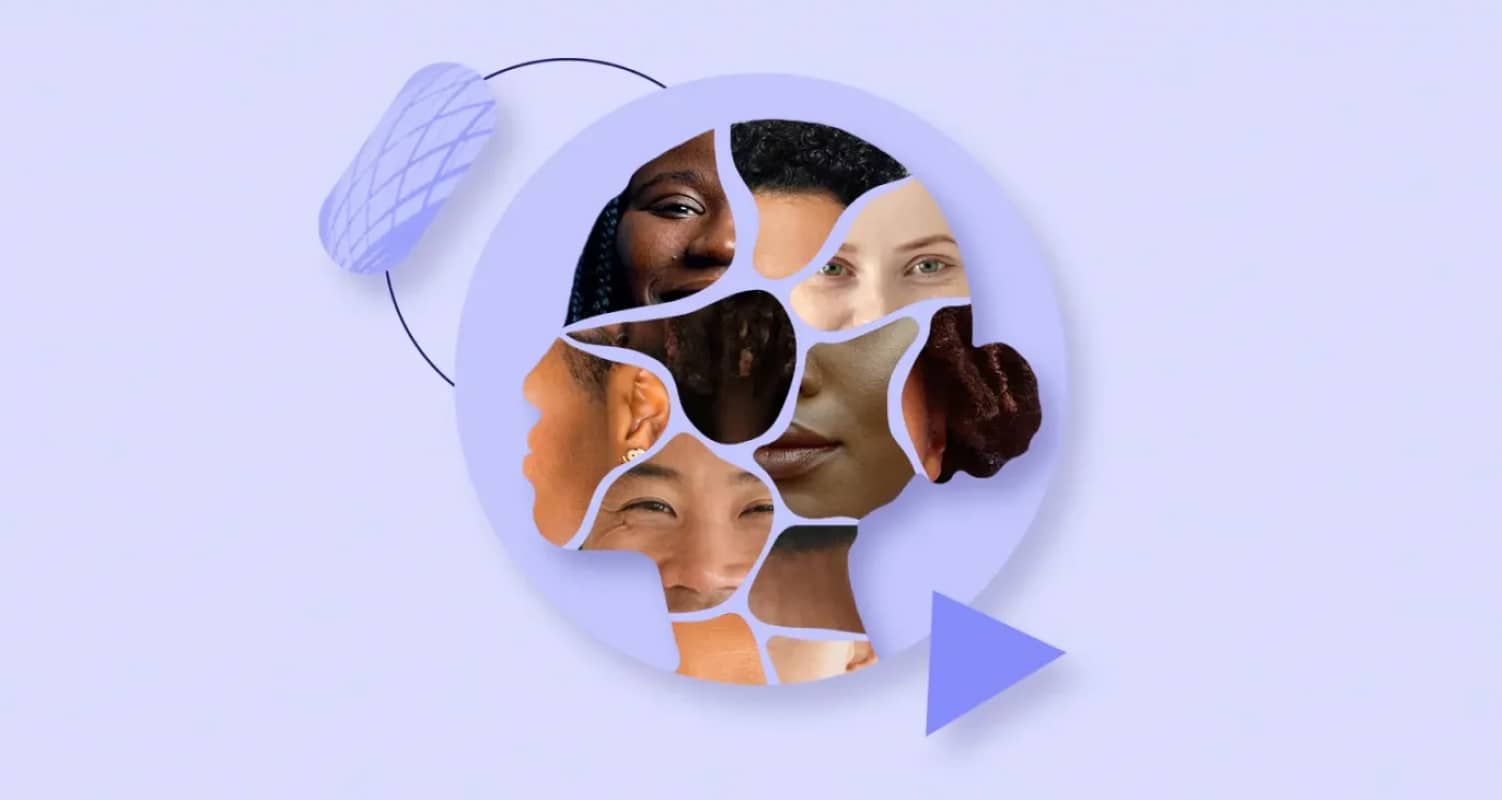
The use of biometrics to verify identity is seeing rapid adoption, particularly facial recognition technology commonly used to unlock smartphones. It's taken time to gain widespread acceptance: for example, when it was newly introduced in Apple iPhones, a study showed that 40% of users in the US said they were unlikely to use facial recognition to authenticate payments.
But now, according to research conducted for our Digital by Default report, people like using biometric verification to open accounts: 8 in 10 respondents found biometrics both secure and convenient. Use of digital identity verification has increased particularly during the last two years as financial services and other businesses have moved online. Facial recognition’s useful applications also include detecting fraudsters, child traffickers and money launderers online, among others.
Biometric verification is powered by artificial intelligence (AI) technology. AI systems are trained with machine learning (ML) models so they know what to recognize and how to categorize and classify what they see. Programmers “feed” the models information to learn from. These datasets provide the examples of what the machine needs to learn, so they need to be representative of reality. That way, the AI learns to assess the same diversity of information that it will likely encounter once it’s operating out in the world.
To be useful, this modern-day facial recognition technology must avoid creating unfair results for some demographic groups due to bias, and should perform the same way for everyone.
Bias in facial recognition technology
Algorithmic bias describes systematic and repeatable errors in an AI system that result in unfair outcomes, such as discriminating against or providing undue advantage to one group of people over another. Algorithmic bias in facial recognition can mean that people in underrepresented demographic groups aren’t accurately recognized, leading to false rejections or false matches during identity verification processes. This can often happen in relation to groups of people with different skin tones.
In fact, bias against correctly recognizing darker skin tones in images goes all the way back to film photography, where light exposure onto film negatives defaulted for lighter skin tones. This bias extended to digital photography and has only recently started to be addressed. In its 2022 Super Bowl campaign video, Google highlighted the accuracy of its Pixel camera technology at depicting the nuances of skin tones. Today, technology is still making progress in solving for fairness and equality in capturing and assessing images.
Where does AI bias come from?
Many things can cause bias: from the design of the algorithm itself to unintended or unanticipated ways of using it, or decisions about what data is used to train the algorithm.
A report from the National Institute of Standards and Technology (NIST) recommends an examination of where AI bias originates, beyond just the data or technology ‘to the broader societal factors that influence how technology is developed.’ The report says that ‘while these computational and statistical sources of bias remain highly important, they do not represent the full picture.’ As such, NIST encourages a ‘socio-technical’ approach to mitigating bias, which Onfido embraces. Socio-technical aspects include privacy, safety, fairness, interpretability and explainability – in other words, context. The sources of bias below include some that are societal, and some that are technical.
Sources of bias
Some categories of bias that can affect AI include systemic bias, statistical or computational bias, and evaluation bias.
-
Systemic bias comes from social constructs. This is primarily human-related, like subjective cultural views or norms, and the decisions we make about people.
-
Statistical or computational bias comes from what is represented in a data set, such as relating to race and gender. For example, white caucasian men are often overrepresented in a data set, which gives an oversimplified view of a more complex reality. That means that when the model encounters white male faces, it will perform very well on facial recognition tasks. Conversely, the algorithm may not perform as well for other, less represented demographics such as people of color or women. This can lead to false matches or false rejections.
-
Evaluation bias is when the statistics of how the model is performing show good performance on overrepresented populations (like white men). It may look like the AI is doing great. But, if the model wasn’t trained enough on women, or people from African countries, for example, those smaller number of cases may have a higher number of false rejections. Thus a high "overall model accuracy" may mean the model performs very successfully on the biggest demographics it has been trained on, while hiding the model's failures on less represented populations.
We believe that algorithms need to operate globally and to the same high standard for everyone. That’s why Onfido takes a multifaceted contextual approach to combating AI bias from its different sources.
What is Onfido doing to mitigate AI bias in digital identity verification?
Inclusivity and accessibility are deeply rooted in Onfido’s culture and we have won awards for our machine learning technology and algorithmic bias mitigation. Onfido’s mission is to power open, secure, and inclusive relationships between businesses and their customers around the world. A dedication to mitigating AI bias is a necessary part of this inclusive mission and is why we worked with the UK’s Information Commissioner’s Office (ICO) to determine a framework for measuring and mitigating it. At Onfido, we examine all the potential sources of bias, not just the technical sources from the data and machine learning models, but from the humans involved as well.
When training the models used in AI, it’s not enough to just throw many data sets into them without care. We give the models diverse and representative data that reflects the reality of the real world.
Then, the models undergo testing, evaluation, validation and verification (TEVV).
How does Onfido test and evaluate our facial recognition AI?
-
We create a balanced test set with diverse, representative examples, that presents all sorts of problems to the model beyond just identifying the most commonly represented Caucasian face. Our AI scientists do data modeling to understand the underlying data distribution, and they may undertake data pre-processing, data augmentation, data labeling and data partitioning among other steps to mitigate bias.
-
While we use metrics that optimize for overall accuracy, that’s not the only important measurement. We look at the differential performance across the classes, so we can see at what point in the lifecycle of the model these biases creep in, and plug the gaps in each place.
-
We do quality control (QC) on the model by monitoring its performance and watching for inevitable data drifts using other specialized AI systems or human monitoring, and make choices about how we react to its performance. If we see a distribution shift in production that causes our false acceptance rate (FAR) to creep up, we make decisions accordingly – for example, do we need to re-train the model on more diverse faces?
-
Our entire governance framework must maintain an anti-bias sensibility. For whatever model we train and deploy, we must identify sources of bias at every stage, and only release it into live use when it passes our standards. We continually watch for bias in production, and retrain the model as new data appears so it continues to learn/improve.
In 2019, Onfido began discussions with the UK ICO and entered its Regulatory Sandbox with the aim of ensuring that the research conducted in relation to improving algorithmic bias was carried out properly. We made certain this vital research respected the rights and freedoms of individuals when processing their personal data.
In line with the objectives outlined in our Sandbox Plan, Onfido’s team trialed and developed methodologies to group and label data, tested the performance of Onfido’s facial recognition, retrained the models, and measured the performance changes to those models.
Onfido gives careful thought to the expectations of all stakeholders - decision makers (customers), end users as well as regulators and compliance functions.
We have made significant investments to develop highly accurate systems, mitigate bias and achieve fairness across demographic groups. This focus on the end-to-end machine learning pipeline led to a 10x reduction in False Acceptance Rates while reducing inter-class differentials in False Rejection Rates.
We undertake fundamental research in face recognition technologies, as demonstrated by our awards, original research publications, (here too), and best-in-class solutions.
Onfido was recognized in the CogX Awards for ‘Best Innovation in Algorithmic Bias Mitigation’ and “Outstanding Leader in Accessibility,’ and was Highly Commended in the SC Europe Awards 2020 for ‘Best Use of Machine Learning.’ The UK magazine Business Leader recognized Onfido in April 2022 as one of the Top 32 AI Companies in the UK.
Discover how to define, measure, and mitigate biometric bias.